Introduction:
Many financial articles are accompanied by line charts or scatter plots that have an overlay or shaded region, as shown in the figure below.The main objective of shading a region is to highlight a particular time in history, or to draw the attention of the reader. In the figure below we see that the highlighted region shows the recession from the fourth quarter of 2007 to the second quarter of 2009.
The dates of recession are published by the National Bureau of Economic Research (NBER). Its actually a good practice to show the recession as it provides reader with a good perspective. For e.g. in the plot above we see that the breakeven inflation fell almost to -1% during financial crisis. So if a drop is observed again sometime after 2009 readers can easily compare. Financial variables and economic indicators exhibited extreme behavior during crisis.
But to create a similar plot in R we need two things knowledge of how to shade regions in R and the dates of recession. So lets come to the real story how do we get business cycle dates for India. I follow a few blogs and one of them is written by Ajay Shah who has written on this topic. The article and business cycle dates for India can be downloaded here.
My aim in this post is to show readers how easy it is to create shaded regions in R using the rect() function.
The plot above shows that GDP in India was not impacted as much by recession of 2008 and 2009. There are many reasons why this is the case and curious minds can google this. We also see that the NBER does not identify any period post 2009 as recessionary phase but we do observe slow down in India economy in the second quarter of 2011.
Packages:
For the purpose of this tutorial we will use two packages WDI and dplyr. The WDI package is used to download the data discussed in the data section. The dplyr package is used for data manipulation and transformation.
install.packages(c("WDI","dplyr"))
library(WDI)
library(dplyr)
options(scipen=999)
The install.packages() function will download the package and library() function will load the library in out current R session. The options(scipen=999) is used to instruct R that i would not like to see data in scientific notation.Data:
We will download the data in R using the WDI package. The data is provided by World Bank via World Development Indicator. To learn more about the indicators, country codes, frequency of data etc, please visit their website. For the purpose of this tutorial we will download the GDP data from 1990 to 2017.## extract GDP data
gdp = WDIsearch(string = "gdp", field = "name", short = TRUE,
cache = NULL)
data = WDI(country = "IN", indicator = "NY.GDP.MKTP.CD",
start = 1990, end = 2017, extra = FALSE, cache = NULL)
The WDIsearch() function is used to search for all the data made available by World Bank. The first argument in this function is a string which can be anything you like search. In our case we would like to search for the GDP data but we could have also replaced string ="gdp" to string ="unemployment" or string="gender". What we would get back is a list of indicators and their names. We need to know the indicator to download the data.
Now to extract the data we will use the WDI() function from WDI package. The first argument is country. In our case we would like the data for India hence "IN" , note that if you like to know the code of your country you can go to the WDI website and get the code or type country ="all" instead of country ="IN". The next argument is the indicator, this where we will use the indicator from the gdp data frame. The start and end date should also be specified.
Data transformation:
I would not like to plot the actual GDP data but somehow shorten the number so that its easily readable on the Yaxis. So we will divide the gdp data by 10^12 and then sort the data.data= mutate(data, gdp = NY.GDP.MKTP.CD/10^12)
data = arrange(data,year)
The mutate() function will create an additional column with the transformed data. the first argument in the mutate function is data, the second argument is the transformation we would like to see. Here, we will divide the data by 10^12. Next , we will arrange the data from 1990 to 2017 using the arrange() function. The first argument in arrange() function is the data and the second argument is the name of column we would like to sort in descending order.
Creating a line chart and adding a shaded region:
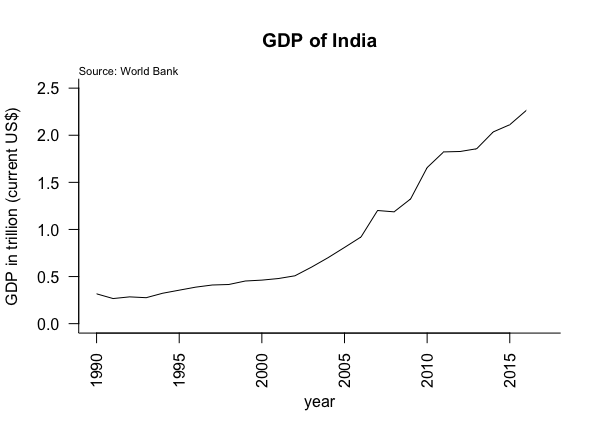
Following line will create a line plot:
plot(data$year,data$gdp, type ="l", las = 2, bty="l",
ylim=c(0,2.5),main = "GDP of India",
ylab = "GDP in trillion (current US$)",
xlab = "year")
In this post the aim is not plot a line chart but create a shaded region. To create a shaded region we need the business cycle dates for India. Now, we know business cycle dates from Ajay Shahs blog. Since, we are only going to plot recession we only need recession dates. India under went recession three time since 1990, following are the recessionary dates:
- 1999 quarter 4 to 2003 quarter 1
- 2007 quarter 2 to 2009 quarter 3
- 2011 quarter 2 to 2012 quarter 4
rect(1999.75,-1,2003.25,2.5,col = rgb(211,211,211,100,max=255), border = FALSE)
rect(2007.50,-1,2009.75,2.5,col = rgb(211,211,211,100,max=255), border = FALSE)
rect(2011.50,-1,2013,2.5,col = rgb(211,211,211,100,max=255), border = FALSE)
the rect() function in R will create a rectangle on the chart. To create a rectangle we usually need the base and the height. similarly, in R the rect() function has four arguments:
The first argument is the xleft, which mean the starting point on the xaxis from where we would like to draw a rectangle. The second argument is ybottom, this is the point on the yaxis. Both the xleft and ybottom will be a point on the plot.The third argument is xright, which is the right most point. So we have the base ready. All we need is a height. The fourth argument is ytop, this is the point on the yaxis corresponding to the xright.
To plot the first recession we will run the following code:
rect(1999.75,-1,2003.25,2.5,col = rgb(211,211,211,100,max=255), border = FALSE)
In the above code the xleft is 1999.75 which is the third quarter of 1999, -1 is used for ybottom. If we do not use -1 the rectangle will have a space between xaxis and base of rectangle. The xright argument is 2003.25 as the recession ends in first quarter of 2003 and finally ytop argument which is 2.5. I picked 2.5 based on the max value of gdp.
We will repeat the above mentioned code for the remaining two recession periods. Finally, we will use the col argument to fill the rectangle with colors and border = FALSE argument will not create a border around the rectangle.
One important point to remember is that the rectangle is created on top of the line chart. Hence, we need to make the rectangle transparent so that we can see the line representing GDP. One very useful trick in R is to use the rgb() function which has an argument for alpha. The alpha argument controls the transparency, i played around with this value till i was happy with the result. To learn more about the rgb() function type ?rgb in R console window.
Conclusion:
In the current post we learned to create a rectangle and overlay it on line plot in R to show recession phases. We see that adding shaded region on the line plot adds context and we can increases the interpretability of a plot.Code:
Please leave a comment if you find issues with the code.library(WDI)
library(dplyr)
options(scipen=999)
## extract GDP data
gdp = WDIsearch(string = "gdp", field = "name", short = TRUE,
cache = NULL)
data = WDI(country = "IN", indicator = "NY.GDP.MKTP.CD",
start = 1990, end = 2017, extra = FALSE, cache = NULL)
## convert data into trillion and arrange it
data= mutate(data, gdp = NY.GDP.MKTP.CD/10^12)
data = arrange(data,year)
#picking color
col.rgb = col2rgb("lightgrey")
print(col.rgb)
## generate the plot
plot(data$year,data$gdp, type ="l", las = 2, bty="l", ylim=c(0,2.5),main = "GDP of India", ylab = "GDP in trillion (current US$)", xlab = "year")
mtext("Source: World Bank",3, adj = 0, outer=FALSE, cex=0.7)
rect(1999.75,-1,2003.25,2.5,col = rgb(211,211,211,100,max=255), border = FALSE)
rect(2007.50,-1,2009.75,2.5,col = rgb(211,211,211,100,max=255), border = FALSE)
rect(2011.50,-1,2013,2.5,col = rgb(211,211,211,100,max=255), border = FALSE)
thanks for sharing this article with valuable points. I am a data analyst and offered data analysis courses in delhi
ReplyDeleteIt is very nice article on that topic. I was looking for something like which is interesting and knowledgeable. Do you know that Agrawal Construction Company has the most amazing townships, especially top Builders In Bhopal, with the name Sagar Green Hills. It is located in the lap of nature.
ReplyDeleteFascinating post. I Have Been considering about this issue, so thankful for posting. Totally cool post.It 's very generally very Useful post.Thanks
ReplyDeletehttps://360digitmg.com/course/certification-program-in-data-science
Really Nice Information It's Very Helpful All courses Checkout Here.
ReplyDeletedata science course in pune
This post is very simple to read and appreciate without leaving any details out. Great work!
ReplyDeleteai course aurangabad
yozgat
ReplyDeletetunceli
hakkari
zonguldak
adıyaman
NL22LG
0414A
ReplyDeleteHatay Parça Eşya Taşıma
Çanakkale Parça Eşya Taşıma
Kastamonu Lojistik
Düzce Parça Eşya Taşıma
Bartın Parça Eşya Taşıma
99100
ReplyDelete%20 indirim kodu
5992B
ReplyDeleteantep en iyi ücretsiz sohbet uygulamaları
yalova seslı sohbet sıtelerı
telefonda kızlarla sohbet
şırnak sohbet siteleri
hatay görüntülü sohbet sitesi
rastgele sohbet
bedava sohbet
bitlis canlı sohbet odası
canlı sohbet odası
16519
ReplyDeleteErgo Coin Hangi Borsada
Bitcoin Nasıl Üretilir
Omlira Coin Hangi Borsada
Tumblr Takipçi Satın Al
Coin Nasıl Alınır
Kwai Beğeni Hilesi
Periscope Beğeni Satın Al
Twitter Takipçi Satın Al
Arg Coin Hangi Borsada
E304C6EFF3
ReplyDeleteinstagram bot takipçi al
6665EB3EF6
ReplyDeleteçekilişle takipçi satın al
M3u Listesi
Raid Promosyon Kodu
Raid Promosyon Kodu
3D Car Parking Para Kodu
Osm Promosyon Kodu
Razer Gold Promosyon Kodu
Erasmus Proje
Happn Promosyon Kodu
5508187C6B
ReplyDeletebot takipçi
beğeni satın al
garantili takipçi
bayan takipçi
aktif takipçi
8CE17AC7F2
ReplyDeletetwitter takipçi satın al
tiktok beğeni satın al
garantili takipçi
düşmeyen takipçi
türk takipçi